🔑 In 10 minutes you will learn:
- The definition and significance of KLUE Benchmark
- The types of Korean language datasets KLUE consist of
- How accurate Datumo’s NLI dataset is, compared to SNLI(Stanford Natural Language Inference) and MNLI(Multi-Genre Natural Language Inference)
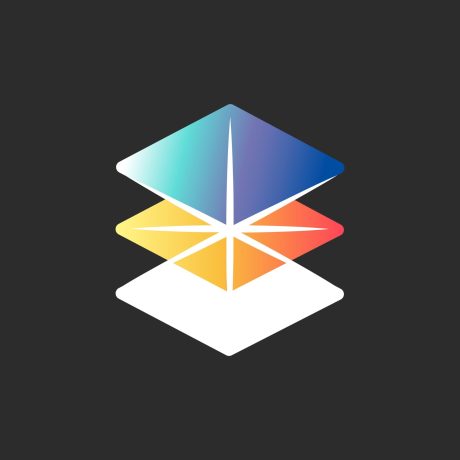
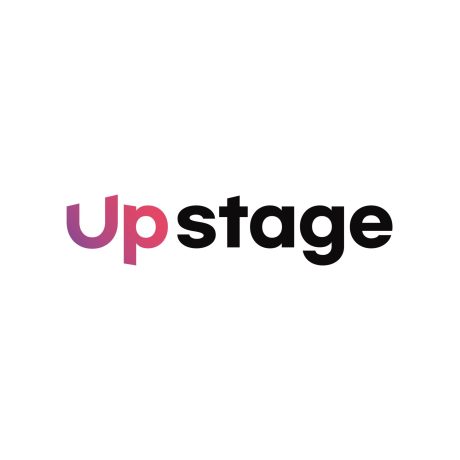
Korean Language Understanding Evaluation Benchmark
Korean
Natural language
NLP
The KLUE(Korean Language Understanding Evaluation Benchmark) paper, written using Datumo’s datasets, has been accepted in NeurIPS 2021, a world-renowned AI conference.
Natural language processing(NLP) has been an on-going research throughout the world. However, there were limitations in utilizing open datasets as the basis for Korean NLP research because most of them were in english, which made it difficult to produce precise results taking the unique characteristics of the Korean language into consideration.
In order to solve this problem, the startup Upstage held hands with 10 other institutes including Korea Advanced Institute of Science and Technology(KAIST), New York University(NYU), Naver, and Google to build KLUE benchmark.
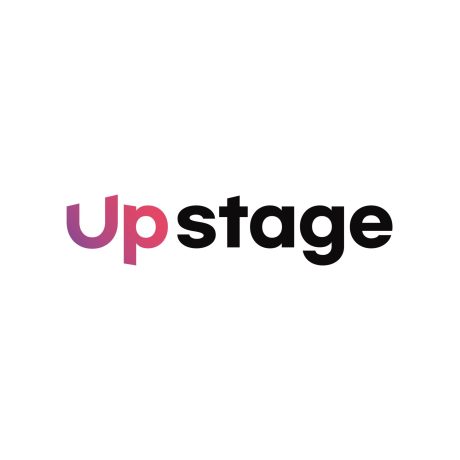
SungJoon Park, Upstage AI research engineer & Chief project manager of Project KLUE
“While building the KLUE dataset with Datumo, we were most impressed by their data quality assurance system. Despite the intricacy of the data and the tight deadline, Datumo was able to provide specific guidelines for the workers to guarantee data consistency. They also made sure to train and select qualified workers, and inspect the entire dataset. We believe that KLUE, the representative Korean NLP benchmark dataset, was able to come into the world, owing to Datumo’s capability and passion.”
Amongst the eight Korean natural language understanding (NLU) tasks, the ones Datumo took care of were as follows:
- Topic Classification
- Semantic Textual Similarity
- Natural Language Inference
- Machine Reading Comprehension.
A number of crowd-workers from Datumo’s crowd-sourcing platform <Cash Mission> swiftly collected and annotated KLUE datasets. We’ll take a further look at the four tasks.
As shown in the photos below, this task required crowd-workers to categorize news headlines into various topics such as politics, economics, society, IT, and more. The categorization does not simply rely on the headline including particular keywords, but on whether the content of the headline is related to certain topic or not.
Once three crowd-workers chose maximum of three topics for each news headline, the headline was categorized as the most-voted topic. In order to maintain data accuracy, workers were also requested to report any headline that includes personally identifiable information (PII), expresses social bias, or is hate speech. The reported headlines were discarded after manual review.
Semantic textual similarity (STS) is necessary to measure the degree of semantic equivalence between two sentences. For this particular task, it was essential to make sure that it is not about monitoring if two sentences had the same exact words used, but about making sure the two sentenced had the same meaning.
“The one that washes well” and “which cleans clothes better” do not have any common vocabulary used, but we know that both mean the same. As such, apart from using the same exact vocabularies, we make sure to compare the speakers’ purpose, nuance, and overall emotion to train AI and make the model smarter.
The purpose of NLI is to train AI models to infer the relationship between a hypothetical sentence and a premise sentence. Datumo’s crowd-workers constructed numerous true/false/neutral sentences based on the premise and classified the sentences made by other workers back as true/false/neutral.
KLUE-NLI, based on Datumo’s data, performed higher accuracy compared to SNLI(Stanford Natural Language Inference) and MNLI(Multi-Genre Natural Language Inference).
Statistics | SNLI | MNLI | KLUE-NLI |
Unanimous Gold Label |
58.30% | 58.20% | 76.29% |
Individual Label = Gold Label |
89.00% | 88.70% | 92.63% |
Individual Label = Author’s Label |
85.80% | 85.20% | 90.92% |
Gold Label = Author’s Label |
91.020% | 92.60% | 96.76% |
Gold Label ≠ Author’s Label |
6.80% | 5.60% | 2.71% |
No Gold Label (No 3 Labels Match) |
2.00% | 1.80% | 0.53% |
We call the label intended by the original annotator in writing the hypothesis “author’s label.” Consensus among three out of five annotators is “gold label.”_Source: KLUE Paper
KLUE’s Machine reading comprehension (MRC) is a task designed to evaluate models’ abilities to read a given text passage and then answer a question about the passage. In order to enable the model to “comprehend” the given text, a number of crowd-workers formulated a pair of sentences(question and answer) and the sentences went through validation.
Text
- According to The Hydrometeorological Centre of Russia, Moscow, known for its cold weather, warmed up to 7 degrees Celsius in the afternoon of22nd(local time).
- Goldman Sachs, an American investment bank and financial services company, have reported on 22nd that the consumption of natural gas and oil for heating is decreasing and that oversupply of oil and hesitance in global economic growth will even lower the cost per unit of energy.
Question
- What was the highest temperature in Moscow on the day Goldman Sachs published their report?
Answer
- 7 degrees Celsius
An AI model trained with KLUE’s MRC dataset should be able to “comprehend” the context and be able to infer an answer for a question. As such, AI is only as smart as the data that feeds it, and that is why achieving high quality data is so crucial.
Dongyeon Kim | Project Manager
“Among the eight KLUE project tasks, Datumo collected and annotated four: TC, STS, NLI, MRC. I was in charge of the MRC task and there were three types within the task.
1. Paraphrasing
2. Multi-sentence
3. Non-answerable
There were some challenges such as setting a standard for acceptable paraphrasing and combining sentences to avoid situations where there could be multiple correct answers. Some text snippets were Korean translations from English, which made some sentences sound unnatural or awkward, whereas some had too many jargons. However, after continuous communication, we were able to come up with a method to maintain the consistency and quality of the final dataset.”
Open Datasets for Data-Centric AI
The above datasets can be downloaded for free through DATUMO ‘OPEN DATASETS’.
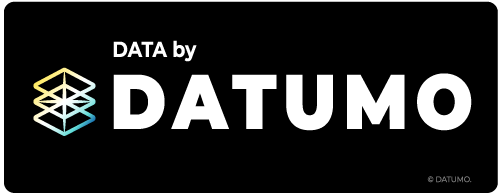
CC BY-SA
Reusers are allowed to distribute, remix, adapt, and build upon the material in any medium or format, even commercially, so long as attribution is given to the creator. If you remix, adapt, or build upon the material, you must license the modified material under identical terms.
https://creativecommons.org/licenses/by-sa/3.0/deed.en